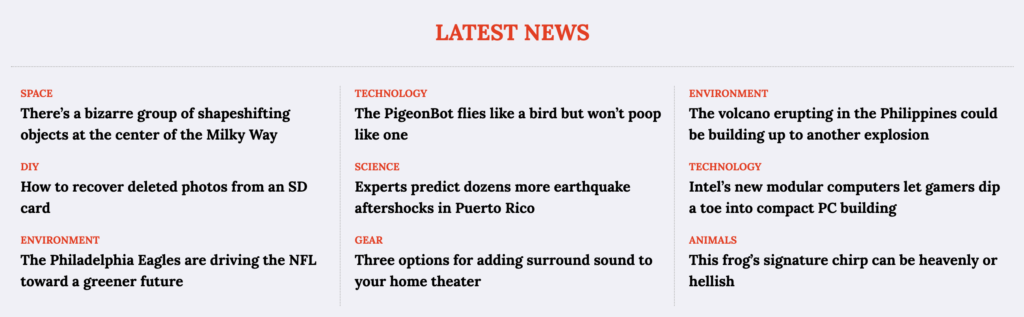
Category Archives: science
Antarctica's doomsday glacier is melting. Can we save it in time?
Read more: https://www.newscientist.com/article/mg24532650-900-antarcticas-doomsday-glacier-is-melting-can-we-save-it-in-time/#ixzz6BW3ve0Lg
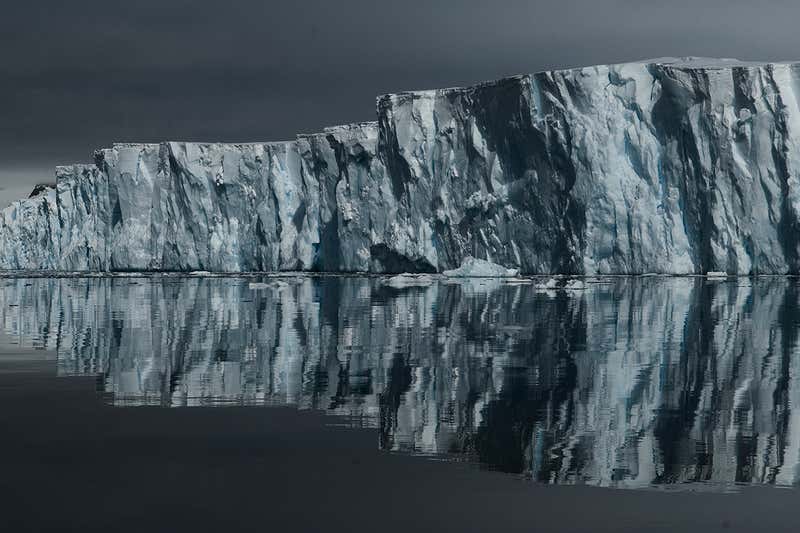
“YOU are very aware that if something goes wrong, it goes very wrong very quickly,” says Joanne Johnson, speaking from her tent near Thwaites glacier in one of the remotest parts of Antarctica. At the time, she and three colleagues were alone, more than 1600 kilometres from the nearest research station. Strong winds had pounded them and it had snowed heavily, making the terrain even more perilous. On the bright side, it was mercifully mild, at -5°C.
Until now, fewer than 50 people have been to this part of West Antarctica, less than have been to space. By the end of this month, 100 will have visited. The reason why is simple: Thwaites is a potential climate time bomb that we need to learn much more about.
This vast glacier is about the size of Great Britain. While it has been shrinking since the early 1990s, ice loss has almost doubled over the past 20 years. It is shedding a dizzying 35 billion tonnes a year. On its own, its collapse would raise seas by around 65 centimetres. That is worrying enough in the context of the 19-cm rise in the whole of the 20th century. But the bigger worry is that this glacier buttresses the entire West Antarctic ice sheet. If Thwaites goes, the fear is it will trigger a wider collapse of ice – enough to raise seas by a calamitous 3.3 metres within a few hundred years.
Seeing around corners: How to decipher shadows to see the invisible
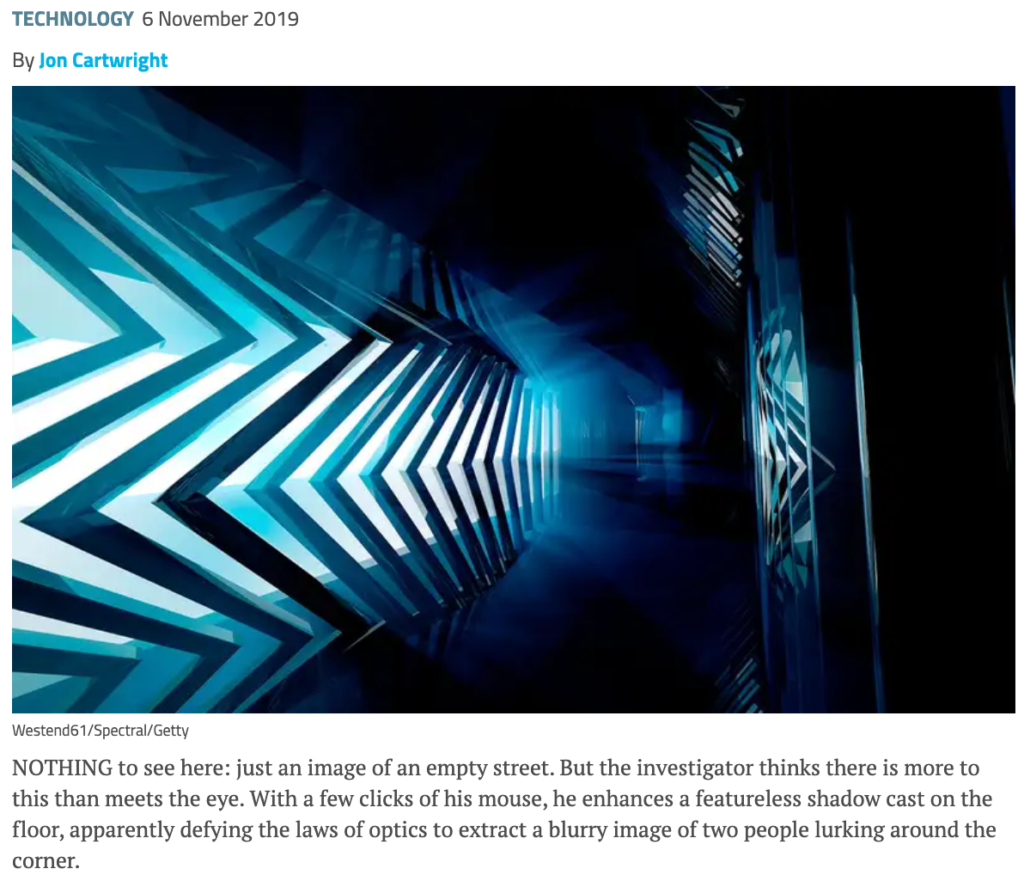
Technical wizardry like this seems far-fetched. But this isn’t CSI. The investigator is a computer scientist not a detective, and those characters are graduate students not suspects. More importantly, this technology is real, and it is being developed in labs right now.
The science of seeing around corners is new, fast-moving and breathtaking. We are discovering that the shadows are full of visual information that our eyes can’t see. Now, as people develop clever ways to make the invisible visible, they are exposing all manner of potential applications besides forensics. Autonomous cars that spot hidden hazards. Cameras that direct fire crews to people trapped in burning buildings. Endoscopes that guide surgery in unreachable parts of the body.
“It could be extremely powerful,” says Vickie Ye, a computer vision researcher at the University of California, Berkeley. “Any information outside the frame could be interpretable.”
You don’t need novel science to see around a corner. You could just use a periscope, or any mirror for that matter. A mirror works because light rays bounce off the surface in a clean and predictable way – namely, at the same angle at which they hit it. As a result, all the visual information collectively contained within the light rays is preserved, …
Planet Earth: Life: S1 Ep8 - Creatures of the Deep
Planet Earth: Creatures of the deep
Planet Earth: Life: Fish
Clownfish Talk Their Way Out of Conflict
Transform Science - Enthought
How flies walk on ceilings
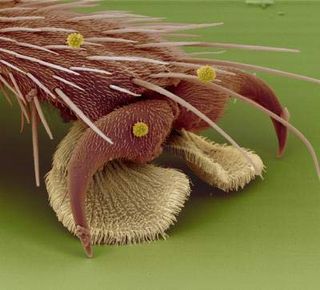
Walking upside-down requires a careful balance of adhesion and weight, and specialized trekking tools to combat the constant tug of gravity.
Each fly foot has two fat footpads that give the insect plenty of surface area with which to cling. The adhesive pads on the feet, called pulvilli, come equipped with tiny hairs that have spatula-like tips. These hairs are called setae.
Scientists once thought that the curved shape of the hairs suggested that flies used them to grip onto the ceiling. In fact, the hairs produce a glue-like substance made of sugars and oils.
Sticky proof
A research team from the German Max Planck Institute for Metals Research recently studied more than 300 species of wall-climbing insects and watched them all leave behind sticky footprints.
|
||
Mystery Monday | ||
Each Monday, this LiveScience series explores an amazing aspect of the world around you. Previous articles: |
- How Viruses Invade Us
- How We Smell
- Why We Lie
- Why Ants Rule the World
- The Science of Traffic Jams
- Why Rice Krispies Go Snap, Crackle, Pop!
- The Shocking Truth Behind Static Electricity
- Why the Ground is Brown
- Why Frogs are Green
- How Dolphins Spin, and Why
"There are over one million insect species," team leader Stanislav Gorb told LiveScience. "We suppose that all of them have the secretion, but it is difficult to be 100 percent sure."
Gorb presented the findings at the Annual Meeting of the Society for Experimental Biology in April.
Flies need sticky feet to walk on ceilings, but not so sticky that they get stuck upside down. So each foot comes with a pair of claws that help hoist the gooey foot off the wall.
Flies use several different techniques to get unstuck: pushing, twisting, and peeling its footpads free.
"Methods involving peeling are always the best, because they require less energy to break the contact," Gorb said.
The combination of the feet hairs' rounded tips, the oily fluid, and a four-feet-on-the-floor rule help the inverted insect take steps in the right direction.
Lessons for robofly
Following in the fly's footsteps, robots are on their way to climbing walls.
Gorb's research team worked with a robotics group from Case Western Reserve University to design robotic feet that mimic a fly's footing.
On the bottom of the feet of a 3-ounce robot that's all legs, scientists tacked on a sticky, furry manmade material that resembles the hairy surface of a fly foot. The researchers also taught the robot how to gently peel its foot off a glass wall, just like a demure insect.
"It's the first time a robot has climbed glass in a way that was inspired by an animal," said mechanical engineer Roger Quinn.
Princeton University: Programming Assignment Checklist: DNA Sequence Alignment
This assignment allows optional partnering. If you choose to do this, you must follow the pair programming guidelines. Please click the link and review them before you begin. Your partner can be from another precept (but ISC students may only partner with other ISC students). Please note that writing code with a partner without following the pair programming instructions is a violation of the course collaboration policy. All writing of code, comments, analysis and uploading to the dropbox should be done together from start to finish. If you come to office hours alone, you can get advice, but you may not change any code until both partners are together.
What are the main goals of this assignment? You will (i) solve a fundamental problem in computational biology, (ii) learn about the analysis of algorithms, and (iii) learn about a powerful programming paradigm known as dynamic programming.
How do I read in the two input strings from the file? Use StdIn.readString() and redirection as usual.
How do I access the length of a string s? The ith character? Use s.length() and s.charAt(i), respectively. As with arrays, indices start at 0. We'll learn about this notation for manipulating (String) objects in Section 3.1. For this assignment, this is all you'll need to know about objects.
Can I assume that the input characters will always be A, C, G or T? NO! Your program should work equally well for any letter, upper case or lower case.
What's a StringIndexOutOfBoundsException? It's just like an ArrayOutOfBoundsException. It results from invoking s.charAt(i) with an illegal value of i.
How could I get a NullPointerException? Did you forget to allocate memory for opt[][]?
How do I declare and initialize a two dimensional array in Java? Review the end of Section 1.4 in Intro to Programming.
It seems strange to define x[i..M] to be the substring consisting of x[i] through x[M-1] instead of x[i] through x[M]. Is this a typo? It's a little strange, but no, it's not a typo. It's consistent with Java's indexing notation where the left endpoint is inclusive and the right endpoint is exclusive.
Which alignment should I print out if there are two or more optimal ones? Output any one you like.
Should gaps be handled by penalty()? The solution we think is clearest does not call penalty() on gaps, since a gap is not a character. Of course, the alignment output should use the '-'symbol to denote a gap, and the inputs we give you will never contain '-' (only alphanumeric characters are used), so if you find it convenient for penalty() to recognize '-' as a gap, you are permitted to do so.
Where can I learn more about dynamic programming and backtracking? The LCS (longest common subsequence) problem described in booksite section 9.6 is an example of a dynamic programming problem on strings with backtracking. However, it is different from the current problem in many ways, so do not simply mimic the code without understanding what it does. The websheet exercises for this week include several dynamic programming exercises starting from a basic level, including KnapsackBacktrack which is an example of backtracking.
What does OutOfMemoryError mean? When Java runs, it requests a certain amount of memory from the operating system. The exact amount depends on the version of Java and your computer, but can vary from 64MB to 1024MB (1GB). After Java has started, the total size of all variables in use cannot be larger than what it originally requested. Trying to do so causes anOutOfMemoryError.
For this assignment, the largest test cases use huge arrays, and Java needs to ask for enough memory from the operating system. To explicitly ask for for more (or less) memory, use the -Xmxflag. For example, to request 500 megabytes (500 MB) of memory for a run, use
java-introcs -Xmx500m EditDistance < input.txt
Here 500m means 500 MB. You should adjust this number depending on the amount of memory your computer has and the size of the arrays you will need for the data set you are running. The amount 500MB should get you through ecoli10000.txt. To run ecoli20000.txt you will need to request more memory. (How much? The readme asks you to estimate this.)
What does "Could not reserve enough space for object heap" mean? This occurs if you use -Xmx with a value that is larger than the amount of available physical memory. Additionally, due to address space limitations, some 32-bit versions of Windows also will give this error if you try to request more than approximately 1.5GB, no matter how much physical memory is installed.
How do I determine how much physical memory is installed on my computer? On Mac, select About this Mac from the Apple menubar. On Windows, press Windows-R (or Run on the Start menu), enter msinfo32
and look for total physical memory.
How can I measure how long my program takes on each file? To measure the running time of your program, there are a few techniques.
- The simplest is to use
java-introcs -Xmx500m EditDistance < input.txt > output.txt
and use a stopwatch. We redirect the output to a file to prevent printing text from becoming a bottleneck.
- A second technique, which we think probably best suits the needs of this application, is to use the -Xprof runtime switch, which asks Java to print out timing data about the run. To use this with output redirection, type
java-introcs -Xprof -Xmx500m EditDistance < input.txt > output.txt
The timing information will appear at the end of the file output.txt, and you want the "flat profile" for main. The line will look like
Flat profile of 4.5 secs (15 total ticks): main
but these numbers are made up and yours will be different. We don't care about the ticks.Piping can be useful here. You can skip the output file in the previous step by piping your output to another program that will look for "Flat profile". and it should print out the time (and throw away all the other program output). On a Mac, run
java-introcs -Xprof -Xmx500m EditDistance < input.txt | grep "main"
On Windows, use find instead of grep:
java-introcs -Xprof -Xmx500m EditDistance < input.txt | find "main"
This find/grep command searches through all of whatever text it is fed and only prints out the lines containing the text main. Type man grep (in Terminal) or find /? (in Command Prompt) for more information.
- As a third technique, you can use Stopwatch objects, see Repeat.java for an example. We'll explain more about objects later in the course.
- Finally, you may elect to directly use System.currentTimeMillis() as shown in lecture.
How do I use a cluster machine in Friend 016/017? See this page for general instructions. Please also read the first bullet point for the question immediately below.
My timing data do not fit a polynomial hypothesis. What could I be doing wrong?
- If you are running your program and accessing the data files from the Windows H: drive (especially if via a wireless network) or ~ on a cluster Mac (Friend 016/017), the bottleneck for medium-sized test cases might be the network latency instead of the dynamic programming algorithm! Do one of the following:
- Use a Stopwatch or System.currentTimeMillis() to specifically isolate the time taken after the input is read (after all calls to StdIn) and before any output is printed. Remember to remove the time printing statements before submitting the final version of your code.
- Or, copy all files to a folder on a local hard drive. We don't recommend this on a cluster (Friend 016/017) machine.
- Or, report your problems and the data you obtained, and use the readme's sample data for analysis instead.
- When you run out of physical memory, your operating system may start using your hard drive as another form of storage. Accessing information from the hard drive is substantially slower than main memory, and you may be observing this effect. Avoid running extraneous complicated programs (media players, file sharing clients, word processors, web browsers, etc) while doing the timing tests if this seems to be a problem.
- Make sure you are using output redirection or piping (as in the examples above) to prevent printing text from becoming a bottleneck.
- Very small test cases are hard to use since the Java virtual machine takes a nontrivial amount of time to start, and since the processor "cache" may make small test cases run an order of magnitude faster than expected. If in doubt, use the test cases that take between 0.1 and 10.0 seconds.
Testing. To help you check the part of your program that generates the alignment, there are many test files in the sequence directory.
- Many of the small files are designed so that it is easy for you to determine what the correct answer should be by hand. Test your program on these cases to see that it gets these easy cases right.
- Here are the optimal edit distances of several of the supplied files.
ecoli2500.txt 118 ecoli5000.txt 160 fli8.txt 6 fli9.txt 4 fli10.txt 2 ftsa1272.txt 758 gene57.txt 8 stx1230.txt 521 stx19.txt 10 stx26.txt 17 stx27.txt 19
- The test case worked through as an example in the assignment description, which is the same as the example10.txt file, has a unique optimal alignment. (Some test inputs like "xx y" have more than one optimal alignment.) So your code should give the exact same output on example10.txt as in the assignment page.
- Here are two more test cases with unique optimal alignments:
% java-introcs EditDistance < endgaps7.txt % java-introcs EditDistance < fli10.txt Edit distance = 4 Edit distance = 2 a - 2 T T 0 t t 0 G G 0 a a 0 G G 0 t t 0 C T 1 t t 0 G G 0 a a 0 G G 0 t t 0 A T 1 - a 2 A A 0 C C 0 T T 0
- In addition, we require that you generate one small input file of your own to be used for testing special cases. Create a new input file with some interesting property. Then test your code using your file and make sure your program behaves as expected. For example, when we tested your RGBtoCMYK program in an earlier assignment, our special case was when R=0, G=0, B=0. In NBody, one of our special cases was a system with only 1 body. Include a description of your special test case in your readme.txt file.
These are purely suggestions for how you might make progress. You do not have to follow these steps.
- Download sequence.zip to your computer and unzip it, as described on the assignment page. It contains test files and the readme templates.
- Write the following two short helper methods.
// return the penalty for aligning character a with character b public static int penalty(char a, char b) // return the min of 3 integers public static int min(int a, int b, int c)
You will call these from your main method to compute penalties and to determine which of the three cases yields the minimum edit distance.
- Write the main() method in EditDistance.java to read in the two strings from standard input, using the method StdIn.readString(). For debugging, print them to standard output.
- Declare and initialize the (M+1)-by-(N+1) array opt[][]. Include the base cases. Print out the 2D array to check your work.To print the matrix out in nicely formatted columns, use
System.out.printf("%3d", opt[i][j]);
with nested for loops. Remember to remove this debugging print section before submitting the final version of your program.
- Now, it's time to implement the crucial dynamic programming part. First read the dynamic programming portion of Section 9.6 and make sure you understand how dynamic programming works. Think carefully about the order in which you will perform the computation since this is critical. Hint: first fill in the base case of opt[i][j], e.g., when i = M or j = N. Now, fill in the remaining values using a nested loop. Test that it works by printing out the contents of opt.
- Now, figure out how to recover the optimal alignment by backtracing.This is an iterative process. At each step we look to see which path choice we should make. Using the example from the assignment we start at i = 0, j=0 where x[i] = 'A' and y[i] = 'T'. The choices are to print "A -" and move down with a gap cost of 2, "- T" and move right with a gap cost of 2, or "A T" and move diagonally with a mismatch cost of 1. We know to pick "A T" because 7 - 6 = 1. This is the only choice which matches the matrix. (It is possible to have more than one choice which matches the matrix. In that case, either choice will lead to the same optimal edit distance.)
Test this part thoroughly. For example, one corner case to test is to make sure that you print out the ENTIRE alignment, even when one sequence finishes before the other. (Use lastXgaps9.txt and lastYgaps9.txt to test.)
- Measure the time that your program takes on the sample runs indicated in the readme. For help on performing timing tests, see above.
- Use the doubling method to estimate the running time as a polynomial function of the input size.
- Does each static method have a comment indicating what it does?
- Are there any hardwired constants? Do they appear in multiple methods? The cleanest solution to this is to use the static class constant
private static int MISMATCH = 1;
(and two other similar ones for gaps and matches). This makes your code easy to use if a different set of penalties is desired.
- The idea of dynamic programming was first advanced by Bellman (1957). Levenshtein (1966) formalized the notion of edit distance. Needleman-Wunsch (1970) were the first to apply edit distance and dynamic programming for aligning biological sequences, and our algorithm is essentially the one proposed in their seminal paper. The widely-used Smith-Waterman(1981) algorithm is quite similar, but solves a slightly different problem (local sequence alignment instead of global sequence alignment).
- The same technology is employed in spell checkers and to identify plagiarism in many courses (including this one).
- The genetic data are taken from GenBank. The National Center for Biotechnology Information also contains many examples of such database and alignment software.
- With a little work, you can compute the optimal cost in quadratic time but using only linear space (do we need the whole
opt
matrix?) With more work, you can also compute the optimal alignment in linear space (and quadratic time). This is known as Hirschberg's algorithm (1975).
Princeton University: Global Sequence Alignment - cos 126
COS 126
Global Sequence Alignment |
Programming Assignment |
This assignment allows optional partnering. If you choose to do this, you must follow the pair programming guidelines. Please click the link and review them before you begin. Your partner can be from another precept (but ISC students may only partner with other ISC students). Please note that writing code with a partner without following the pair programming instructions is a violation of the course collaboration policy. All writing of code, comments, analysis and uploading to the dropbox should be done together from start to finish. If you come to office hours alone, you can get advice, but you may not change any code until both partners are together.
Write a program to compute the optimal sequence alignment of two DNA strings. This program will introduce you to the field of computational biology in which computers are used to do research on biological systems. Further, you will be introduced to a powerful algorithmic design paradigm known as dynamic programming.
Biology review. A genetic sequence is a string formed from a four-letter alphabet {Adenine (A), Thymine (T), Guanine (G), Cytosine (C)} of biological macromolecules referred to together as the DNA bases. A gene is a genetic sequence that contains the information needed to construct a protein. All of your genes taken together are referred to as the human genome, a blueprint for the parts needed to construct the proteins that form your cells. Each new cell produced by your body receives a copy of the genome. This copying process, as well as natural wear and tear, introduces a small number of changes into the sequences of many genes. Among the most common changes are the substitution of one base for another and the deletion of a substring of bases; such changes are generally referred to as point mutations. As a result of these point mutations, the same gene sequenced from closely related organisms will have slight differences.
The problem. Through your research you have found the following sequence of a gene in a previously unstudied organism.
A A C A G T T A C C
What is the function of the protein that this gene encodes? You could begin a series of uninformed experiments in the lab to determine what role this gene plays. However, there is a good chance that it is a variant of a known gene in a previously studied organism. Since biologists and computer scientists have laboriously determined (and published) the genetic sequence of many organisms (including humans), you would like to leverage this information to your advantage. We'll compare the above genetic sequence with one which has already been sequenced and whose function is well understood.
T A A G G T C A
If the two genetic sequences are similar enough, we might expect them to have similar functions. We would like a way to quantify "similar enough."
Edit-distance. In this assignment we will measure the similarity of two genetic sequences by their edit distance, a concept first introduced in the context of coding theory, but which is now widely used in spell checking, speech recognition, plagiarism detection, file revisioning, and computational linguistics. We align the two sequences, but we are permitted to insert gaps in either sequence (e.g., to make them have the same length). We pay a penalty for each gap that we insert and also for each pair of characters that mismatch in the final alignment. Intuitively, these penalties model the relative likeliness of point mutations arising from deletion/insertion and substitution. We produce a numerical score according to the following table, which is widely used in biological applications:
operation cost insert a gap 2 align two characters that mismatch 1 align two characters that match 0
Here are two possible alignments of the strings x = "AACAGTTACC" and y = "TAAGGTCA":
x y cost ------------ A T 1 A A 0 C A 1 A G 1 G G 0 T T 0 T C 1 A A 0 C - 2 C - 2 --- 8 x y cost ------------ A T 1 A A 0 C - 2 A A 0 G G 0 T G 1 T T 0 A - 2 C C 0 C A 1 --- 7
The first alignment has a score of 8, while the second one has a score of 7. The edit-distance is the score of the best possible alignment between the two genetic sequences over all possible alignments. In this example, the second alignment is in fact optimal, so the edit-distance between the two strings is 7. Computing the edit-distance is a nontrivial computational problem because we must find the best alignment among exponentially many possibilities. For example, if both strings are 100 characters long, then there are more than 10^75 possible alignments.
We will explain a recursive solution which is an elegant approach. However it is far too inefficient because it recalculates each subproblem over and over. Once we have defined the recursive definition we can redefine the solution using a dynamic programming approach which calculates each subproblem once.
A recursive solution. We will calculate the edit-distance between the two original strings x and y by solving many edit-distance problems on smaller suffixes of the two strings. We use the notation x[i] to refer to character i of the string. We also use the notation x[i..M] to refer to the suffix of x consisting of the characters x[i], x[i+1], ..., x[M-1]. Finally, we use the notation opt[i][j] to denote the edit distance of x[i..M] and y[j..N]. For example, consider the two strings x = "AACAGTTACC" and y = "TAAGGTCA" of length M = 10 and N = 8, respectively. Then, x[2] is 'C', x[2..M] is "CAGTTACC", and y[8..N] is the empty string. The edit distance of x and y is opt[0][0].
Now we describe a recursive scheme for computing the edit distance of x[i..M] and y[j..N]. Consider the first pair of characters in an optimal alignment of x[i..M] with y[j..N]. There are three possibilities:
- The optimal alignment matches x[i] up with y[j]. In this case, we pay a penalty of either 0 or 1, depending on whether x[i] equals y[j], plus we still need to align x[i+1..M] with y[j+1..N]. What is the best way to do this? This subproblem is exactly the same as the original sequence alignment problem, except that the two inputs are each suffixes of the original inputs. Using our notation, this quantity is opt[i+1][j+1].
- The optimal alignment matches the x[i] up with a gap. In this case, we pay a penalty of 2 for a gap and still need to align x[i+1..M] with y[j..N]. This subproblem is identical to the original sequence alignment problem, except that the first input is a proper suffix of the original input.
- The optimal alignment matches the y[j] up with a gap. In this case, we pay a penalty of 2 for a gap and still need to align x[i..M] with y[j+1..N]. This subproblem is identical to the original sequence alignment problem, except that the second input is a proper suffix of the original input.
The key observation is that all of the resulting subproblems are sequence alignment problems on suffixes of the original inputs. To summarize, we can compute opt[i][j] by taking the minimum of three quantities:
opt[i][j] = min { opt[i+1][j+1] + 0/1, opt[i+1][j] + 2, opt[i][j+1] + 2 }
This equation works assuming i < M and j < N. Aligning an empty string with another string of length k requires inserting k gaps, for a total cost of 2k. Thus, in general we should set opt[M][j] = 2(N-j) and opt[i][N] = 2(M-i). For our example, the final matrix is:
| 0 1 2 3 4 5 6 7 8 x\y | T A A G G T C A - ----------------------------------- 0 A | 7 8 10 12 13 15 16 18 20 1 A | 6 6 8 10 11 13 14 16 18 2 C | 6 5 6 8 9 11 12 14 16 3 A | 7 5 4 6 7 9 11 12 14 4 G | 9 7 5 4 5 7 9 10 12 5 T | 8 8 6 4 4 5 7 8 10 6 T | 9 8 7 5 3 3 5 6 8 7 A | 11 9 7 6 4 2 3 4 6 8 C | 13 11 9 7 5 3 1 3 4 9 C | 14 12 10 8 6 4 2 1 2 10 - | 16 14 12 10 8 6 4 2 0
By examining opt[0][0], we conclude that the edit distance of x and y is 7.
A dynamic programming approach. A direct implementation of the above recursive scheme will work, but it is spectacularly inefficient. If both input strings have N characters, then the number of recursive calls will exceed 2^N. To overcome this performance bug, we use dynamic programming. (Read the first section of Section 9.6 for an introduction to this technique.) Dynamic programming is a powerful algorithmic paradigm, first introduced by Bellman in the context of operations research, and then applied to the alignment of biological sequences by Needleman and Wunsch. Dynamic programming now plays the leading role in many computational problems, including control theory, financial engineering, and bioinformatics, including BLAST (the sequence alignment program almost universally used by molecular biologists in their experimental work). The key idea of dynamic programming is to break up a large computational problem into smaller subproblems, store the answers to those smaller subproblems, and, eventually, use the stored answers to solve the original problem. This avoids recomputing the same quantity over and over again. Instead of using recursion, use a nested loop that calculates opt[i][j] in the right order so that opt[i+1][j+1], opt[i+1][j], and opt[i][j+1] are all computed before we try to compute opt[i][j].
Recovering the alignment itself. The above procedure describes how to compute the edit distance between two strings. We now outline how to recover the optimal alignment itself. The key idea is to retrace the steps of the dynamic programming algorithm backwards, re-discovering the path of choices (highlighted in red in the table above) from opt[0][0] to opt[M][N]. To determine the choice that led to opt[i][j], we consider the three possibilities:
- The optimal alignment matches x[i] up with y[j]. In this case, we must have opt[i][j] = opt[i+1][j+1] if x[i] equals y[j], or opt[i][j] = opt[i+1][j+1] + 1 otherwise.
- The optimal alignment matches x[i] up with a gap. In this case, we must have opt[i][j] = opt[i+1][j] + 2.
- The optimal alignment matches y[j] up with a gap. In this case, we must have opt[i][j] = opt[i][j+1] + 2.
Depending on which of the three cases apply, we move diagonally, down, or right towards opt[M][N], printing out x[i] aligned with y[j] (case 1), x[i] aligned with a gap (case 2), or y[j]aligned with a gap (case 3). In the example above, we know that the first T aligns with the first A because opt[0][0] = opt[1][1] + 1, but opt[0][0] ≠ opt[1][0] + 2 and opt[0][0] ≠ opt[0][1] + 2. The optimal alignment is:
x y cost ------------ A T 1 A A 0 C - 2 A A 0 G G 0 T G 1 T T 0 A - 2 C C 0 C A 1
API specification. Your program EditDistance.java must be organized as a library of static methods with the following API:
public class EditDistance -------------------------------------------------------------------------------- int penalty(char a, char b) // return the penalty for aligning char a and char b int min(int a, int b, int c) // return the min of 3 integers void main(String[] args) // read 2 strings from standard input. // compute and print the edit distance between them. // output an optimal alignment and associated penalties.
Your program. Write a program EditDistance.java that reads, from standard input, two strings of characters. (Although, in the application described, the characters represent genetic sequences, your program should handle any sequence of alphanumeric characters.) Your program should then compute and print the edit distance between the two strings. Finally, it should recover the optimal alignment and print it out along with the individual penalties, using the following format:
- The first line should contain the edit distance, preceded by the text "Edit distance = ".
- Each subsequent line should contain a character from the first string, followed by the paired character from the second string, followed by the associated penalty. Use the character '-'to indicate a gap in either string.
Here is a sample execution:
% java-introcs EditDistance < example10.txt Edit distance = 7 A T 1 A A 0 C - 2 A A 0 G G 0 T G 1 T T 0 A - 2 C C 0 C A 1
The .zip file for this week contains short test data files and actual genomic data files, as well as the readme.txt for this assignment (recall the unzipping instructions here). Or, you can use thesequence directory course ftp site.
Be sure to test thoroughly using the short test files and the longer actual data files. Also, make up a short test file of your own and describe it in your readme.txt file.
Analysis. After you have tested your program using not only the example provided above, but also the many short test data files in the sequence subdirectory, it is time to analyze its running time and memory usage. Using the genomic data sets referred to in the readme.txt file, use the doubling method to estimate the running time (in seconds) of your program as a function of the lengths of the two input strings M and N. For simplicity, assume M = N in your analysis. Also analyze the memory usage (in bytes). Be sure to enter these results in your readme and answer all the questions.
See the checklist for information about giving Java more memory and running timing tests.
Submission. One partner should submit the files EditDistance.java and readme.txt (including the analysis and test data you created). If you are partnering, the second partner should only submit this abbreviated partner readme.txt.
This assignment was created by Thomas Clarke, Robert Sedgewick, Scott Vafai and Kevin Wayne.
Copyright © 2002.How Flies Walk on Ceilings
How Flies Walk on Ceilings
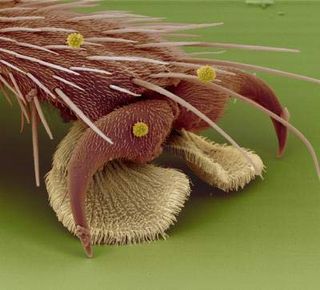
Walking upside-down requires a careful balance of adhesion and weight, and specialized trekking tools to combat the constant tug of gravity.
Each fly foot has two fat footpads that give the insect plenty of surface area with which to cling. The adhesive pads on the feet, called pulvilli, come equipped with tiny hairs that have spatula-like tips. These hairs are called setae.
Scientists once thought that the curved shape of the hairs suggested that flies used them to grip onto the ceiling. In fact, the hairs produce a glue-like substance made of sugars and oils.
Sticky proof
A research team from the German Max Planck Institute for Metals Research recently studied more than 300 species of wall-climbing insects and watched them all leave behind sticky footprints.
|
||
Mystery Monday | ||
Each Monday, this LiveScience series explores an amazing aspect of the world around you. Previous articles: |
- How Viruses Invade Us
- How We Smell
- Why We Lie
- Why Ants Rule the World
- The Science of Traffic Jams
- Why Rice Krispies Go Snap, Crackle, Pop!
- The Shocking Truth Behind Static Electricity
- Why the Ground is Brown
- Why Frogs are Green
- How Dolphins Spin, and Why
"There are over one million insect species," team leader Stanislav Gorb told LiveScience. "We suppose that all of them have the secretion, but it is difficult to be 100 percent sure."
Gorb presented the findings at the Annual Meeting of the Society for Experimental Biology in April.
Flies need sticky feet to walk on ceilings, but not so sticky that they get stuck upside down. So each foot comes with a pair of claws that help hoist the gooey foot off the wall.
Flies use several different techniques to get unstuck: pushing, twisting, and peeling its footpads free.
"Methods involving peeling are always the best, because they require less energy to break the contact," Gorb said.
The combination of the feet hairs' rounded tips, the oily fluid, and a four-feet-on-the-floor rule help the inverted insect take steps in the right direction.
Lessons for robofly
Following in the fly's footsteps, robots are on their way to climbing walls.
Gorb's research team worked with a robotics group from Case Western Reserve University to design robotic feet that mimic a fly's footing.
On the bottom of the feet of a 3-ounce robot that's all legs, scientists tacked on a sticky, furry manmade material that resembles the hairy surface of a fly foot. The researchers also taught the robot how to gently peel its foot off a glass wall, just like a demure insect.
"It's the first time a robot has climbed glass in a way that was inspired by an animal," said mechanical engineer Roger Quinn.
Live Science is part of Future plc, an international media group and leading digital publisher. Visit our corporate site.
Through the Wormhole
A daily journal of 3000 pics a day! How to process and select.
https://www.youtube.com/channel/UCVRl6Rj03lfF8v5nX7eJwyA
![]() |
Through the Wormhole - Top Documentary Films All episodes from Through the Wormhole series gathered in one place. Hosted by Morgan Freeman, Through the Wormhole will explore the deepest mysteries of... |
Source: topdocumentaryfilms.com/through-the-wormhole/ |
Ocean Deep
The Seasonal Forest
Michio Kaku (2014) "Coming Breakthroughs" [FULL SPEECH]
Dr. Michi Kaku
The World in 2030 by Dr. Michio Kaku
Lattice light sheet microscopy
Google Science Fair
PU School of Engineering and Applied Science
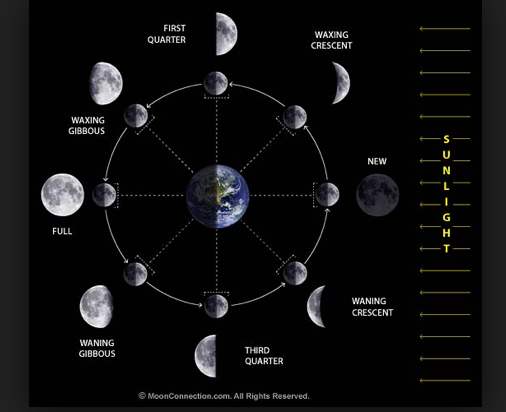